数据分析之美 决策树R语言实现
【摘要】数据分析之美:决策树R语言实现R语言实现决策树1 准备数据[plain] view plain copy > install packages("tree") > library(tree)
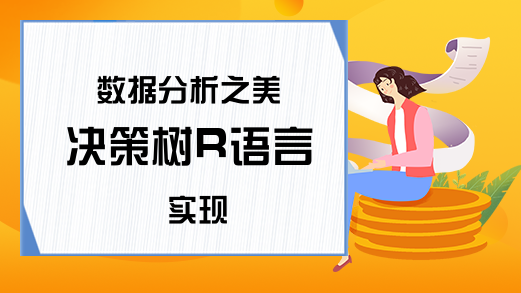
数据分析之美:决策树R语言实现
R语言实现决策树
1.准备数据
[plain] view plain copy
> install.packages("tree")
> library(tree)
> library(ISLR)
> attach(Carseats)
> High=ifelse(Sales<=8,"No","Yes") //set high values by sales data to calssify
> Carseats=data.frame(Carseats,High) //include the high data into the data source
> fix(Carseats)
2.生成决策树
[plain] view plain copy
> tree.carseats=tree(High~.-Sales,Carseats)
> summary(tree.carseats)
[plain] view plain copy
//output training error is 9%
Classification tree:
tree(formula = High ~ . - Sales, data = Carseats)
Variables actually used in tree construction:
[1] "ShelveLoc" "Price" "Income" "CompPrice" "Population"
[6] "Advertising" "Age" "US"
Number of terminal nodes: 27
Residual mean deviance: 0.4575 = 170.7 / 373
Misclassification error rate: 0.09 = 36 / 400
3. 显示决策树
[plain] view plain copy
> plot(tree . carseats )
> text(tree .carseats ,pretty =0)
4.Test Error
[plain] view plain copy
//prepare train data and test data
//We begin by using the sample() function to split the set of observations sample() into two halves, by selecting a random subset of 200 observations out of the original 400 observations.
> set . seed (1)
> train=sample(1:nrow(Carseats),200)
> Carseats.test=Carseats[-train,]
> High.test=High[-train]
//get the tree model with train data
> tree. carseats =tree (High~.-Sales , Carseats , subset =train )
//get the test error with tree model, train data and predict method
//predict is a generic function for predictions from the results of various model fitting functions.
> tree.pred = predict ( tree.carseats , Carseats .test ,type =" class ")
> table ( tree.pred ,High. test)
High. test
tree. pred No Yes
No 86 27
Yes 30 57
> (86+57) /200
[1] 0.715
5.决策树剪枝
[plain] view plain copy
/**
Next, we consider whether pruning the tree might lead to improved results. The function cv.tree() performs cross-validation in order to cv.tree() determine the optimal level of tree complexity; cost complexity pruning is used in order to select a sequence of trees for consideration.
For regression trees, only the default, deviance, is accepted. For classification trees, the default is deviance and the alternative is misclass (number of misclassifications or total loss).
We use the argument FUN=prune.misclass in order to indicate that we want the classification error rate to guide the cross-validation and pruning process, rather than the default for the cv.tree() function, which is deviance.
If the tree is regression tree,
> plot(cv. boston$size ,cv. boston$dev ,type=’b ’)
*/
> set . seed (3)
> cv. carseats =cv. tree(tree .carseats ,FUN = prune . misclass ,K=10)
//The cv.tree() function reports the number of terminal nodes of each tree considered (size) as well as the corresponding error rate(dev) and the value of the cost-complexity parameter used (k, which corresponds to α.
> names (cv. carseats )
[1] " size" "dev " "k" " method "
> cv. carseats
$size //the number of terminal nodes of each tree considered
[1] 19 17 14 13 9 7 3 2 1
$dev //the corresponding error rate
[1] 55 55 53 52 50 56 69 65 80
$k // the value of the cost-complexity parameter used
[1] -Inf 0.0000000 0.6666667 1.0000000 1.7500000
2.0000000 4.2500000
[8] 5.0000000 23.0000000
$method //miscalss for classification tree
[1] " misclass "
attr (," class ")
[1] " prune " "tree. sequence "
[plain] view plain copy
//plot the error rate with tree node size to see whcih node size is best
> plot(cv. carseats$size ,cv. carseats$dev ,type=’b ’)
/**
Note that, despite the name, dev corresponds to the cross-validation error rate in this instance. The tree with 9 terminal nodes results in the lowest cross-validation error rate, with 50 cross-validation errors. We plot the error rate as a function of both size and k.
*/
> prune . carseats = prune . misclass ( tree. carseats , best =9)
> plot( prune . carseats )
> text( prune .carseats , pretty =0)
//get test error again to see whether the this pruned tree perform on the test data set
> tree.pred = predict ( prune . carseats , Carseats .test , type =" class ")
> table ( tree.pred ,High. test)
High. test
tree. pred No Yes
No 94 24
Yes 22 60
> (94+60) /200
[1] 0.77
就业培训申请领取

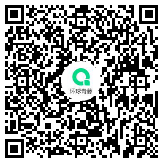
环球青藤
官方QQ群扫描上方二维码或点击一键加群,免费领取大礼包,加群暗号:青藤。 一键加群
数据分析师相关文章推荐
|数据分析师最新文章推荐
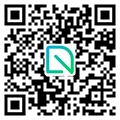
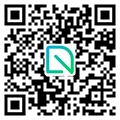
刷题看课 APP下载
免费直播 一键购课
代报名等人工服务
- 1 传统数据和大数据的区别
- 2 数据分析的8种方法都是哪些?
- 3 3大常用的数据分析工具是什么?
- 4 数据分析的具体流程是什么?
- 5 excel中的运算符
- 6 数据分析的作用有哪些?
- 7 电子商务需要分析哪些数据?
- 8 数据分析包括哪些内容?
- 9 rdd是什么?
- 10 数据分析的基本步骤